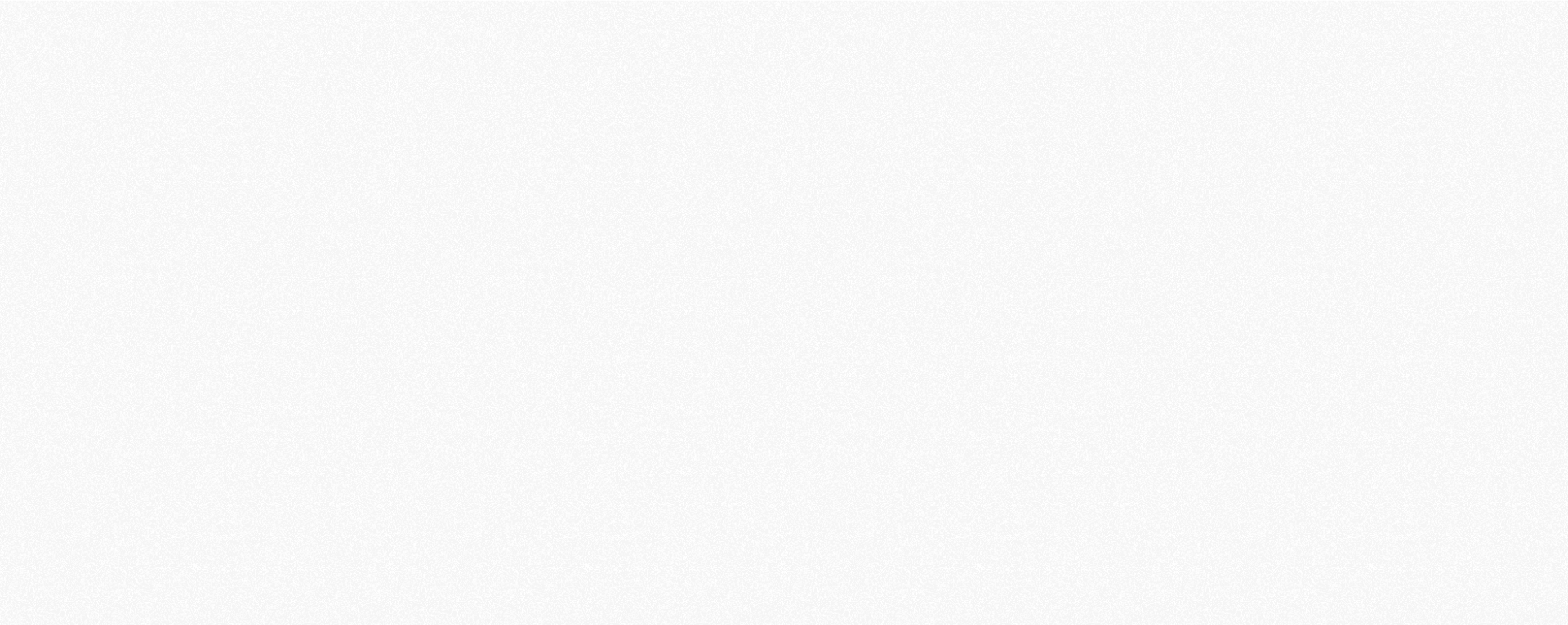
Artificial Intelligence in Image Recognition: Architecture and Examples
Image Recognition in Artificial Intelligence Future of Image Recognition
Convolutional Neural Networks (CNNs) enable deep image recognition by using a process called convolution. These algorithms process the image and extract features, such as edges, textures, and shapes, which are then used to identify the object or feature. Image recognition technology is used in a variety of applications, such as self-driving cars, security systems, and image search engines. Massive amounts of data is required to prepare computers for quickly and accurately identifying what exactly is present in the pictures. Some of the massive databases, which can be used by anyone, include Pascal VOC and ImageNet. They contain millions of keyword-tagged images describing the objects present in the pictures – everything from sports and pizzas to mountains and cats.
This smart system uses photo recognition and to improve its accuracy our software engineers keep training it. The developers upload a sample photo, actually dozens or even hundreds of them and let the system explore the digital image, detect what car is on it, what kind of damage is present, what parts are broken, etc. Thoroughly pre trained system can detect and provide all information within seconds and make the work of insurance agents more effective, fast and accurate. Security means a lot, that is why it is important for companies ensuring it to go hand in hand with advanced technologies and cutting edge devices.
Data Science Trends: Wisdom for Aspiring Data Scientists Advice for Aspiring Data Scientists
In today’s article you will learn how image recognition AI works and how Vistex uses AI and image recognition. In other words, the engineer’s expert intuitions and the quality of the simulation tools they use both contribute to enriching the quality of these Generative Design algorithms and the accuracy of their predictions. Figure 2 shows an image recognition system example and illustration of the algorithmic framework we use to apply this technology for the purpose of Generative Design. Such systems can be installed in the hallways or on devices to prevent strangers from entering the building or using any company data stored on the devices.
With so much online conversation happening through images, it’s a crucial digital marketing tool. After the image is broken down into thousands of individual features, the components are labeled to train the model to recognize them. The first max-pooling layer’s output was condensed to 128×497 and 128×997 pixels. In succeeding layers, same procedures are repeated with various filter sizes.
Product searches through image recognition software
Image Recognition gives computers the ability to identify objects, people, places, and texts in any image. The typical neural networks stack the original image into a list and turn it to be the input layer. In contrast, CNN’s constructs the convolution layer that retains the information between neighboring pixels.
- Annotations for segmentation tasks can be performed easily and precisely by making use of V7 annotation tools, specifically the polygon annotation tool and the auto-annotate tool.
- One of the more promising applications of automated image recognition is in creating visual content that’s more accessible to individuals with visual impairments.
- With the capability to process vast amounts of visual data swiftly and accurately, it outshines manual methods, saving time and resources.
- The varieties available will ensure that the model predicts accurate results when tested on sample data.
- With cameras equipped with motion sensors and image detection programs, they are able to make sure that all their animals are in good health.
This paper reviews basic issues in medical imaging and neural network-based systems for medical image interpretation. In the framework of intelligent systems, a simple scheme that has been implemented is presented as an example of the use of intelligent systems to discriminate between normal and cancerous regions in colonoscopic images. Preliminary results indicate that this scheme is capable of high accuracy detection of abnormalities within the image. It can also be successfully applied to different types of images, to detect abnormalities that belong to different cancer types. Different aspects of education industries are improved using deep learning solutions. Currently, online education is common, and in these scenarios, it isn’t easy to track the reaction of students using their webcams.
It can be big in life-saving applications like self-driving cars and diagnostic healthcare. But it also can be small and funny, like in that notorious photo recognition app that lets you identify wines by taking a picture of the label. Improvements made in the field of AI and picture recognition for the past decades have been tremendous.
- The sheer scale of the problem was too large for existing detection technologies to cope with.
- Moreover, it excels at few-shot learning, achieving impressive results on large image datasets like ImageNet with only a handful of labeled examples.
- Today’s vehicles are equipped with state-of-the-art image recognition technologies enabling them to perceive and analyze the surroundings (e.g. other vehicles, pedestrians, cyclists, or traffic signs) in real-time.
- Essentially, you’re cleaning your data ready for the AI model to process it.
- Every iteration of simulations or tests provides engineers with new learning on how to best refine their design, based on complex goals and constraints.
- Such systems can be installed in the hallways or on devices to prevent strangers from entering the building or using any company data stored on the devices.
Detecting text is yet another side to this beautiful technology, as it opens up quite a few opportunities (thanks to expertly handled NLP services) for those who look into the future. Recurrent Neural Networks (RNNs) are a type of neural network designed for sequential data analysis. They possess internal memory, allowing them to process sequences and capture temporal dependencies.
How to Use Data Cleansing & Data Enrichment to Improve Your CRM
Read more about https://www.metadialog.com/ here.
4 Charts That Show Why AI Progress Is Unlikely to Slow Down – TIME
4 Charts That Show Why AI Progress Is Unlikely to Slow Down.
Posted: Wed, 02 Aug 2023 07:00:00 GMT [source]